Phase 3 of Microsoft’s Secure by Default framework expands auto-labeling to all data in your tenant, using smarter classifiers, simulation, and feedback loops.
Extending Protection with Auto-Labeling and Custom Classifiers
So far in this series, we’ve covered an overview of Microsoft’s Secure by Default framework and examined the Foundational and Managed phases. These initial phases focused on securing new data and high-value locations using Microsoft Purview sensitivity labels and DLP policies.
Now, in Phase 3: Optimized, the focus shifts to extending protection to the remaining data at rest across the tenant. This phase introduces:
- Client-side and service-side auto-labeling
- Advanced use of Sensitive Information Types (SITs)
- Trainable classifiers
- Customization and tuning for better accuracy
This article assumes you’re already familiar with Purview Sensitivity Labels, Data Loss Prevention (DLP) policies, and the configuration principles from earlier phases. We also assume you’ve scoped these policies to a pilot group before full deployment—an essential best practice.
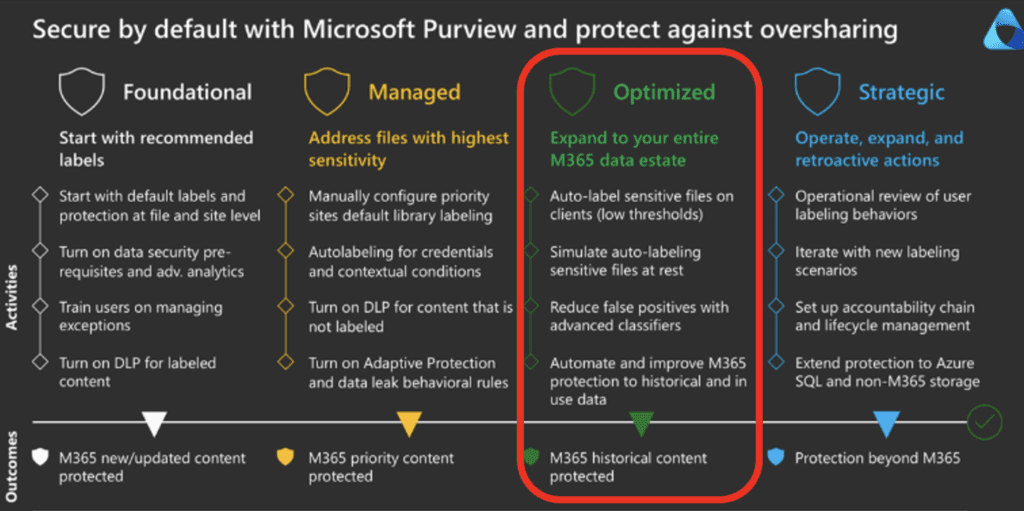
Quick Recap: What’s Already in Place (Phases 1 & 2)
Capability | Status |
---|---|
Default label for new content | Applied as Confidential / All Employees |
Default label on sensitive libraries | Applied to protect known critical content |
DLP blocking unlabeled items | Enforced across email and files |
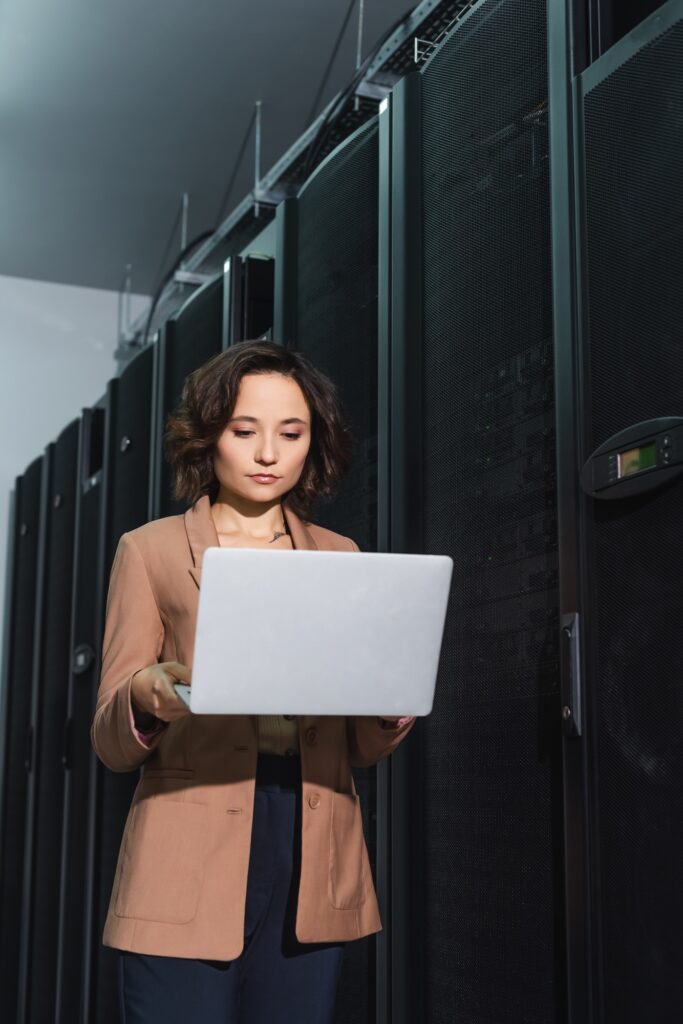
Phase 3 Objectives and Actions
1. Enable Client-Side Auto-Labeling for New or Edited Content
Apply stricter labels when content contains sensitive information at the endpoint level.
How to do it:
- Identify SITs or Trainable Classifiers that require stronger protection than your default label.
- Configure a sensitivity label policy that either recommends or enforces stricter labeling when these types are detected.
Expected Outcome:
- Users will be prompted to apply stricter labels (or have them auto-applied) when working with PII, credentials, or regulated content.
- Staff familiarity from previous phases will reduce friction and support faster adoption.
- Users retain control when appropriate, overriding labels if needed.
Use this alongside effective training to ensure users understand when and why labels change automatically.
2. Apply Service-Side Auto-Labeling for Data at Rest
Label existing files based on content using server-side automation.
How to do it:
- Define SITs, thresholds (e.g., number of instances), and scope (SharePoint, OneDrive, Exchange).
- Run the labeling policy in simulation mode for tuning before enabling enforcement.
Expected Outcome:
- Coverage can expand as classifiers improve in accuracy.
- Documents with sensitive data are labeled more precisely than using library-level defaults.
- Protection adapts to content, not just location.
- Users may notice changes to file labels—communicate proactively to manage expectations.
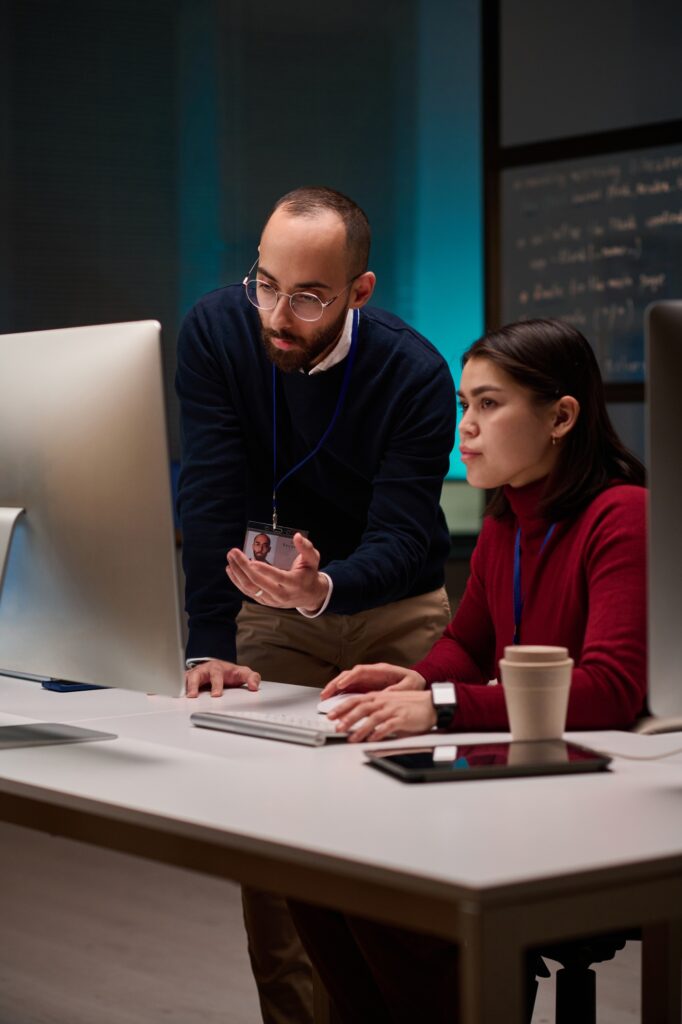
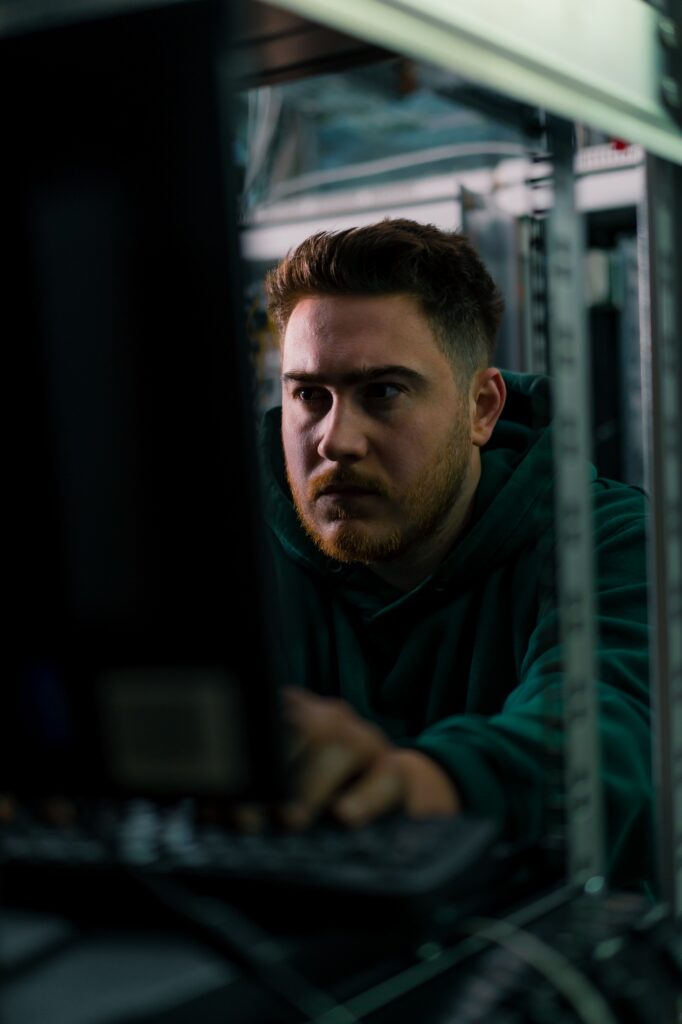
3. Refine SITs and Develop Custom Classifiers
Improve detection accuracy and reduce false positives by optimizing your classification methods.
How to do it:
- Review user feedback and simulation results from auto-labeling.
- Customize or clone built-in SITs; consider EDM-based SITs or Fingerprinting for niche data types.
- Build Trainable Classifiers for unstructured or unique content patterns (this takes time—plan accordingly).
- Use combinations of SITs, confidence levels, and classifiers to fine-tune DLP and labeling policies.
Expected Outcome:
- Classification accuracy improves, reducing user frustration and support overhead.
- Enables expanded adoption of Microsoft Purview features like Insider Risk Management or Data Lifecycle Management.
- Boosts incident response readiness with more precise controls.
Tip: Document classifier logic and confidence thresholds to improve policy governance and help desks.
Keep Feedback Loops Open
As your labeling automation expands, ongoing feedback is crucial. Encourage pilot users to report issues, and establish a process to quickly triage and address them.
In some cases, you may need to temporarily exclude certain users or groups if workflows are disrupted—just ensure that long-term plans align with Secure by Default principles.
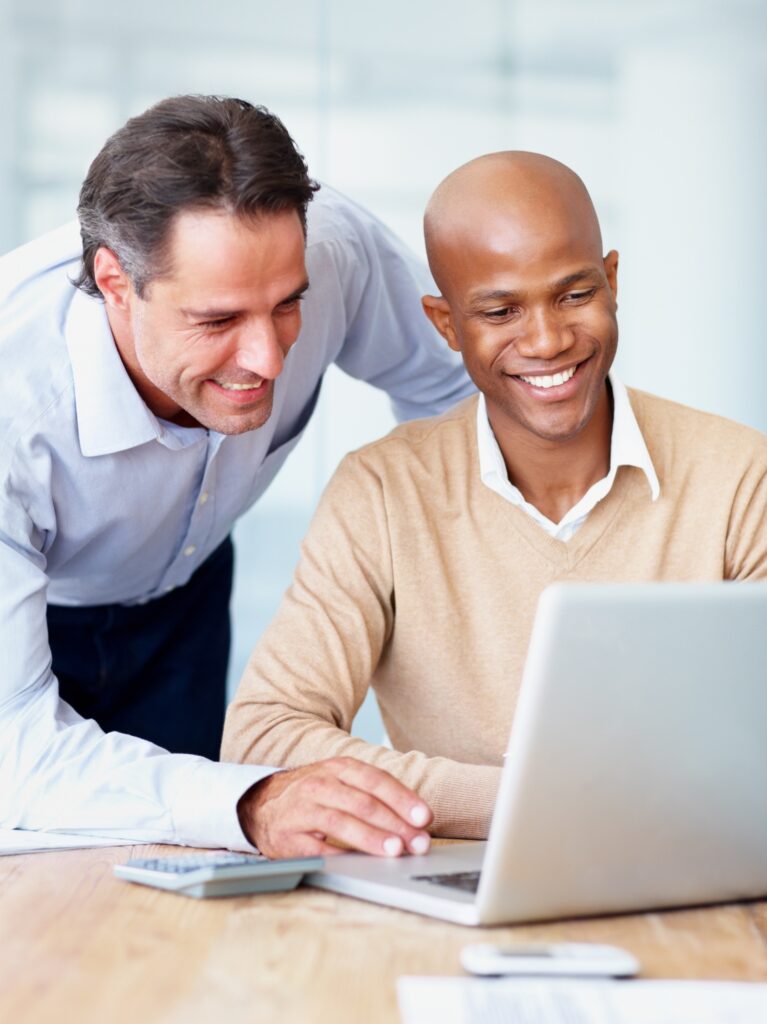
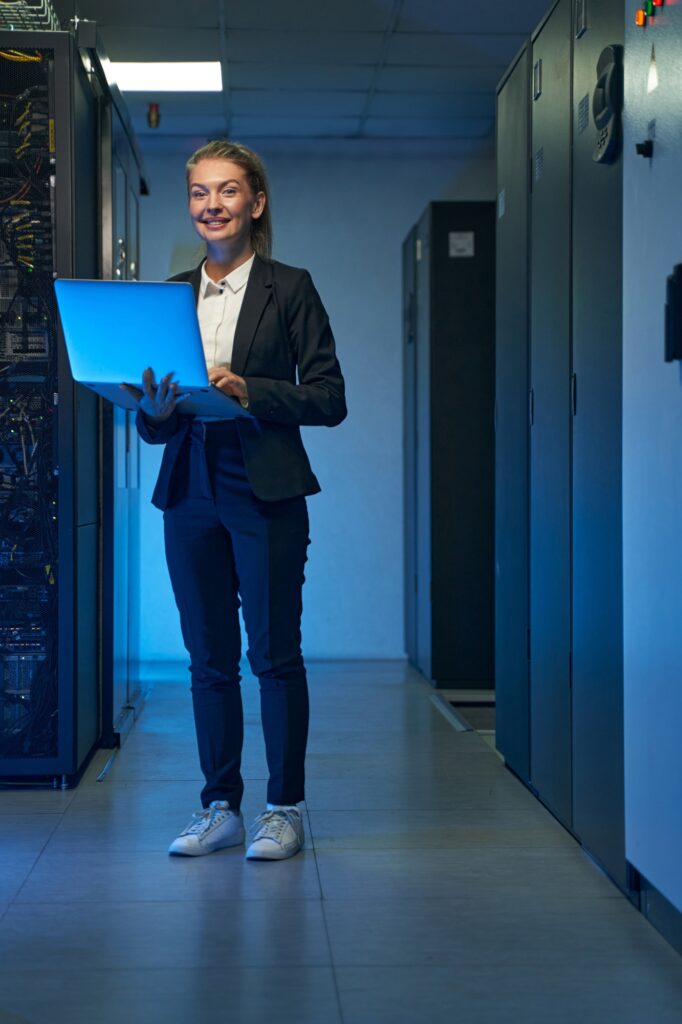
What’s Next?
At this stage, Microsoft Purview Information Protection and DLP should cover most or all of your M365 tenant.
But this is far from the finish line. Future refinements include:
- Adding granular labels and policy conditions
- Extending labeling to data outside M365 (e.g., in cloud storage or on-premises systems)
Stay tuned for the next installment of this series, where we’ll dive into Phase 4: Strategic, and explore how to align Secure by Default with long-term data governance.
Need Help Implementing the Secure by Default Framework with Purview?
Whether you’re fine-tuning auto-labeling, building custom classifiers, or scaling your Microsoft 365 information protection strategy, eGroup can help.
Our experts have hands-on experience guiding organizations through every phase of Microsoft Purview Secure by Default–from foundational configuration to optimized automation.
Let’s secure your data estate with confidence.
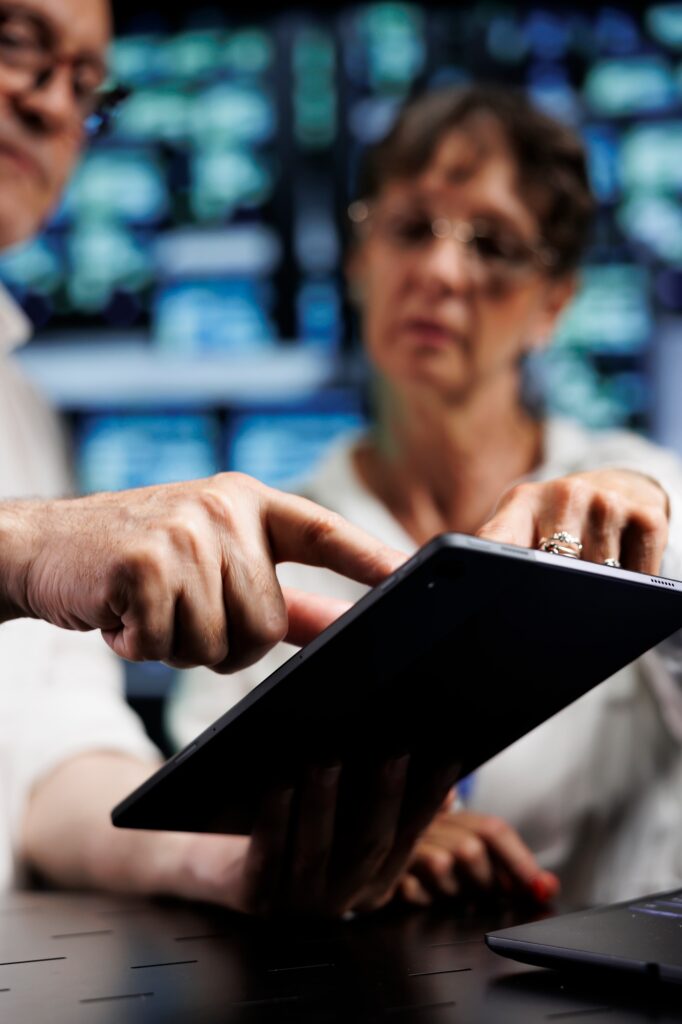